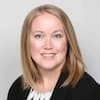
Casting a Wide Talent Net and Finding Phenomenal Talent With Machine Learning
There are many other reasons that candidates don’t apply. I would recommend getting feedback from applicants and going through the process yourself. There are also a number of companies that will audit your candidate experience. We offer a mini audit at no cost but your career site does have to meet some qualifications.
Having a lot of candidates for a job sounds like a good thing but most recruiters will run away from the idea because they don't have the right tools to be able to accurate pick out the cream of the crop. To address this, companies are implementing new ways of rating active and passive candidates using machine learning.
Casting a Wide Talent Net
The best candidates are not always ones that apply on their own. To get the best pool of talent, you need to capture both active and passive candidates.
On average, only 4% of career site visitors apply for a job. Why? We did audits on 400 of the fortune 1000 in our State of Talent Relationship Marketing Report and found a few commons issues that will impact your visit to apply rate.
Capturing Passive Candidates
What about the window shoppers? This is where you can get the most amount of leads for a job but how do you identify people that haven’t applied? One great way we have found is giving job seekers the ability to log in through their LinkedIn account and then capturing their information. This allows you to contact them to start a conversion or start nurturing them to get them to apply. By the way, nurturing can be 100% automated. Nurturing should also be used to stay in touch with candidates for future openings. Even if a candidate isn’t the right fit for your company, they can still refer their connections.
Selecting the Cream of the Crop with Machine Learning
Machine learning is a hot topic right now and is being developed and applied to most industries. So what is it? Simply put, machine learning is a type of Artificial Intelligence that provides computers with the ability to learn on its own by analyzing data. This means the more data it can analyze, the smarter it gets.
Machine learning is being applied to recruiting and talent acquisition on multiple fronts from providing a personalized candidate experience to increase conversions to candidate “fit” rating. While rating candidates based on keywords in their resume can be helpful, it’s only as smart as the person entering the keywords and the person writing the resume. Machine learning can rate based on whatever data you can gather. Career sites are starting to capture data on user activity, which can help rate a candidate’s interest level but you can go far beyond that. Feedback on why a candidate was hired or even not hired can be thrown into the mix to find commonalities with other candidates. This is just the start of what will change recruiting and talent acquisition in the near future.
The Challenge with Machine Learning and Applying it to Recruiting and Talent Acquisition
Machine learning sounds great but why isn’t it widely used in recruiting and talent acquisition today? You need a lot of data (applicants) for machine learning to be accurate enough to provide good ratings and recommendations. If there is a sufficient amount data from candidates, machine learning is still only based on what you know about a candidate like their work history, activity on your career site and responses to emails. So, you need to also capture data and feedback on the recruiter and hiring manager side, again you need a lot of data to make predictions accurate.
Interested in casting a wide talent net and finding phenomenal talent with machine learning? Learn more about the Phenom People TRM Cloud Platform or get a Demo.
Get the latest talent experience insights delivered to your inbox.
Sign up to the Phenom email list for weekly updates!